The Core Truths to Maximize Value of Data
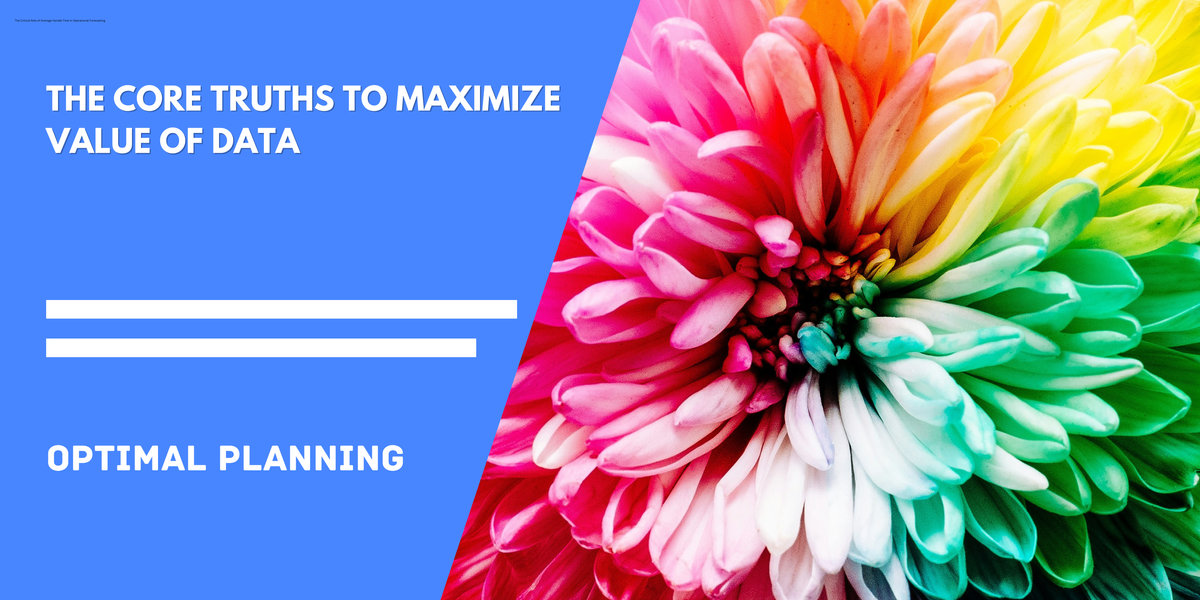
As the amount of data available in a contact center grows over time, there are almost infinite ways to analyze and report on trends. As a data enthusiast, I enjoy diving deep to uncover insights and answer pressing questions about business performance. Here are some core truths I constantly remind myself to maximize the value of data and minimize its pitfalls:
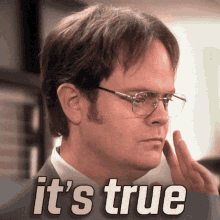
First, there is no perfect data. Every raw data point starts off being recorded in an unstructured way. It is up to the data engineer to transform this unstructured data into structured data suitable for analytics. The transformation process is largely influenced by the engineer's and stakeholders' expectations. Therefore, it's impossible for all structured data to meet everyone's needs. Accepting that there is no perfect data and understanding its inner logic allows you to provide context, pros, and cons when explaining insights to stakeholders.
Second, value the "what" over the "why." Analytics professionals often hypothesize why certain business metrics trend in specific ways. While understanding the context behind the metrics is important, the real value lies in deciding "What are we going to do to improve this metric?" Businesses that act on data insights will outperform those that merely speculate and navel gaze.
Third, consolidation is key. Many organisations have numerous data dashboards, with more than majority of being infrequently used. Periodically evaluating and consolidating these data dashboards can save resources and time, ensuring that only the most useful tools are maintained. By consolidating data metrics to the ones that truly move performance makes it easier for the business to rally around improving the metric.
Fourth, while composite metrics are useful, simple metrics are often better. Composite metrics, which combine several sub-metrics, can obscure the drivers behind the overall metric. By breaking down and reporting on individual sub-metrics, it becomes easier for operational leaders to understand the data. For example, the S&P 500 index tracks the 500 largest companies and is heavily influenced by the "Magnificent 7" stocks (Apple, Microsoft, Amazon, Alphabet, Meta, Nvidia, and Tesla). While the S&P 500 is up about 23% in the past year, excluding these seven stocks shows an increase of only about 7%. This demonstrates the importance of looking beyond composite metrics to understand true performance drivers.
Finally, data is not static. While we might want data to remain consistent for year-over-year comparisons, this is not practical. As businesses evolve, so do the underlying data and measurement methodologies. It's crucial to understand when significant changes in data methodologies occur and provide context to stakeholders when presenting data.
Effective data analysis requires embracing the imperfections of data, prioritizing actionable insights over mere speculation, consolidating redundant data dashboards, simplifying metrics, and acknowledging the dynamic nature of data. By adhering to these principles, you can unlock the potential of data to drive business success.