Building a Capacity Confidence Matrix for Better Business Planning
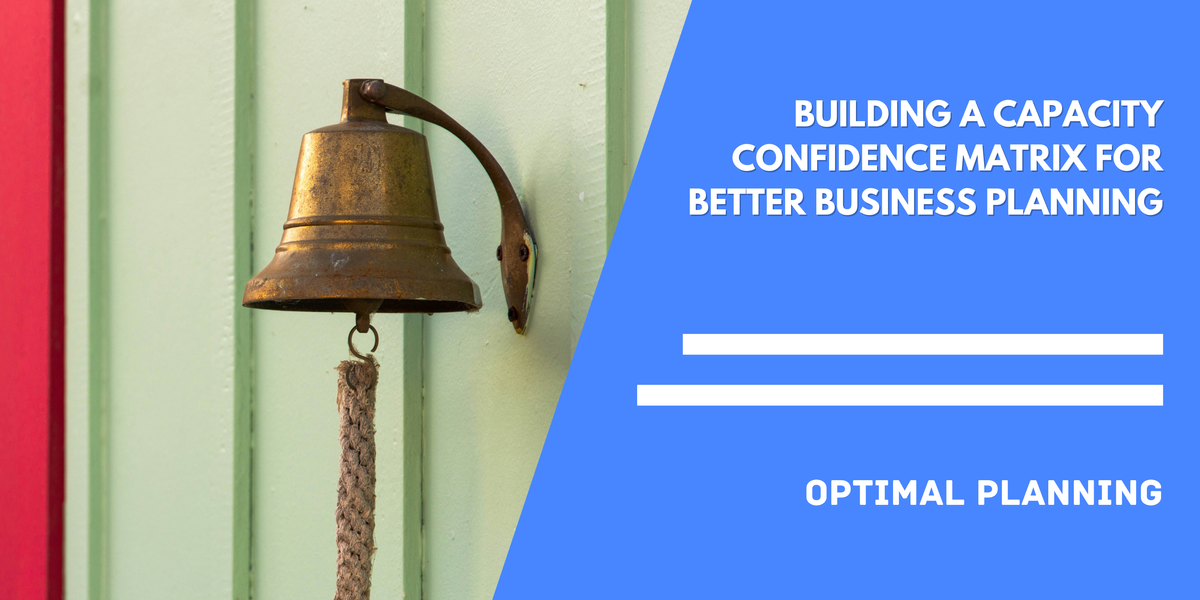
I've previously written about the importance of planning for a range of outcomes, suggesting that one should create multiple scenarios to understand how capacity would fare if certain inputs, such as demand, shrinkage, attrition, and other factors, change. This approach allows capacity planners to scenario-plan and inform the business about conditions under which capacity is in good shape and when it is not. However, I didn't delve into how to quantify the probability of these capacity outcomes. Here, I will share a simple process that I like to use to quickly quantify the likelihood of capacity success or failure.
First, let's cover a bit of statistics—don't worry, it won't be too intense. For example, when you graph the daily average handling time (AHT) or daily volume on a distribution graph or histogram, it shows how often these daily stats fall into specific ranges. Most of the time, this data will form a normal distribution, which looks like a bell curve. In a normal distribution, most values cluster around the central value, with fewer occurrences at the extremes. The area under the graph represents all the possibilities the call center has experienced over the range of dates from which the data was pulled.
For instance, if you plot daily AHT, you might see the left tail of the graph representing days with extremely low AHT (perhaps days when systems were down and associates could only tell clients they would get back to them). As you move right, the graph rises, representing the number of days closer to BAU conditions. The peak of the graph represents the most common AHT values. Further right, the graph falls, representing days with extremely high AHT (possibly days when associates handled complex, unusual inquiries without standard procedures).
To calculate the cumulative percentage of AHT (i.e., the number of days with AHT below a specific value), you sum all the days with AHT below that value and divide by the total number of daily observations.
Why is this important? By assigning a probability to whether your capacity will be in good shape, you can make informed decisions. For example, if you choose an AHT at the 85th percentile and your capacity plan still shows sufficient capacity, there's a high likelihood that the contact center's capacity is in good shape for the future.
You can apply the same logic to other KPIS such as demand and shrinkage to estimate the probability of capacity being adequate. You can also combine several KPIs into a matrix to create a capacity confidence table.
There are, however, a few caveats to this type of range thinking:
1. Historical Values: Historical values are just that—historical. As the business moves forward, it will encounter days outside the historical range, so you can't be 100% confident in the probability calculated from past data.
2. Seasonality: Seasonality impacts confidence levels. If you only consider historical values from non-peak seasons and apply that to a capacity plan for peak season, your AHT assumptions will be off and so will the probability you assign to it.
3. Growth Rate: Growth rate also impacts confidence. If the business launches more complex products, the historical AHT values may no longer be accurate, as the future range of possible AHT will likely shift upwards.
By creating a probability table on multiple ranges of KPI outcomes it has helped me gain confidence when I am projecting a call center’s capacity. However, it's also important to realize that any system has flaws as I outlined above and one shouldn’t use this one method to assign probabilities of success. Experience and business qualitative feedback is also critical to help calibrate your confidence level in your capacity plan.